Summary
-------
Databases come in a variety of formats for different use cases. The default association with the term "database" is relational engines, but non-relational engines are also used quite widely. In this episode Oren Eini, CEO and creator of RavenDB, explores the nuances of relational vs. non-relational engines, and the strategies for designing a non-relational database.
Announcements
-------------
---Hello and welcome to the Data Engineering Podcast, the show about modern data management
---This episode is brought to you by Datafold – a testing automation platform for data engineers that prevents data quality issues from entering every part of your data workflow, from migration to dbt deployment. Datafold has recently launched data replication testing, providing ongoing validation for source-to-target replication. Leverage Datafold's fast cross-database data diffing and Monitoring to test your replication pipelines automatically and continuously. Validate consistency between source and target at any scale, and receive alerts about any discrepancies. Learn more about Datafold by visiting [dataengineeringpodcast.com/datafold] (https://www.dataengineeringpodcast.com/datafold) .
---Dagster offers a new approach to building and running data platforms and data pipelines. It is an open-source, cloud-native orchestrator for the whole development lifecycle, with integrated lineage and observability, a declarative programming model, and best-in-class testability. Your team can get up and running in minutes thanks to Dagster Cloud, an enterprise-class hosted solution that offers serverless and hybrid deployments, enhanced security, and on-demand ephemeral test deployments. Go to [dataengineeringpodcast.com/dagster] (https://www.dataengineeringpodcast.com/dagster) today to get started. Your first 30 days are free!
---Data lakes are notoriously complex. For data engineers who battle to build and scale high quality data workflows on the data lake, Starburst powers petabyte-scale SQL analytics fast, at a fraction of the cost of traditional methods, so that you can meet all your data needs ranging from AI to data applications to complete analytics. Trusted by teams of all sizes, including Comcast and Doordash, Starburst is a data lake analytics platform that delivers the adaptability and flexibility a lakehouse ecosystem promises. And Starburst does all of this on an open architecture with first-class support for Apache Iceberg, Delta Lake and Hudi, so you always maintain ownership of your data. Want to see Starburst in action? Go to [dataengineeringpodcast.com/starburst] (https://www.dataengineeringpodcast.com/starburst) and get $500 in credits to try Starburst Galaxy today, the easiest and fastest way to get started using Trino.
---Your host is Tobias Macey and today I'm interviewing Oren Eini about the work of designing and building a NoSQL database engine
Interview
---------
---Introduction
---How did you get involved in the area of data management?
---Can you describe what constitutes a NoSQL database?
------How have the requirements and applications of NoSQL engines changed since they first became popular ~15 years ago?
---What are the factors that convince teams to use a NoSQL vs. SQL database?
------NoSQL is a generalized term that encompasses a number of different data models. How does the underlying representation (e.g. document, K/V, graph) change that calculus?
---How have the evolution in data formats (e.g. N-dimensional vectors, point clouds, etc.) changed the landscape for NoSQL engines?
---When designing and building a database, what are the initial set of questions that need to be answered?
------How many "core capabilities" can you reasonably design around before they conflict with each other?
---How have you approached the evolution of RavenDB as you add new capabilities and mature the project?
------What are some of the early decisions that had to be unwound to enable new capabilities?
---If you were to start from scratch today, what database would you build?
---What are the most interesting, innovative, or unexpected ways that you have seen RavenDB/NoSQL databases used?
---What are the most interesting, unexpected, or challenging lessons that you have learned while working on RavenDB?
---When is a NoSQL database/RavenDB the wrong choice?
---What do you have planned for the future of RavenDB?
Contact Info
------------
--- [Blog] (https://ayende.com/blog/)
--- [LinkedIn] (https://www.linkedin.com/in/ravendb/?originalSubdomain=il)
Parting Question
----------------
---From your perspective, what is the biggest gap in the tooling or technology for data management today?
Closing Announcements
---------------------
---Thank you for listening! Don't forget to check out our other shows. [Podcast.__init__] (https://www.pythonpodcast.com) covers the Python language, its community, and the innovative ways it is being used. The [Machine Learning Podcast] (https://www.themachinelearningpodcast.com) helps you go from idea to production with machine learning.
---Visit the [site] (https://www.dataengineeringpodcast.com) to subscribe to the show, sign up for the mailing list, and read the show notes.
---If you've learned something or tried out a project from the show then tell us about it! Email [hosts@dataengineeringpodcast.com] (mailto:hosts@dataengineeringpodcast.com) ) with your story.
Links
-----
--- [RavenDB] (https://ravendb.net/)
--- [RSS] (https://en.wikipedia.org/wiki/RSS)
--- [Object Relational Mapper (ORM)] (https://en.wikipedia.org/wiki/Object%E2%80%93relational_mapping)
--- [Relational Database] (https://en.wikipedia.org/wiki/Relational_database)
--- [NoSQL] (https://en.wikipedia.org/wiki/NoSQL)
--- [CouchDB] (https://couchdb.apache.org/)
--- [Navigational Database] (https://en.wikipedia.org/wiki/Navigational_database)
--- [MongoDB] (https://www.mongodb.com/)
--- [Redis] (https://redis.io/)
--- [Neo4J] (https://neo4j.com/)
--- [Cassandra] (https://cassandra.apache.org/_/index.html)
--- [Column-Family] (https://en.wikipedia.org/wiki/Column_family)
--- [SQLite] (https://www.sqlite.org/)
--- [LevelDB] (https://github.com/google/leveldb)
--- [Firebird DB] (https://firebirdsql.org/)
--- [fsync] (https://man7.org/linux/man-pages/man2/fsync.2.html)
--- [Esent DB?] (https://learn.microsoft.com/en-us/windows/win32/extensible-storage-engine/extensible-storage-engine-managed-reference)
--- [KNN == K-Nearest Neighbors] (https://en.wikipedia.org/wiki/K-nearest_neighbors_algorithm)
--- [RocksDB] (https://rocksdb.org/)
--- [C# Language] (https://en.wikipedia.org/wiki/C_Sharp_(programming_language))
--- [ASP.NET] (https://en.wikipedia.org/wiki/ASP.NET)
--- [QUIC] (https://en.wikipedia.org/wiki/QUIC)
--- [Dynamo Paper] (https://www.allthingsdistributed.com/files/amazon-dynamo-sosp2007.pdf)
--- [Database Internals] (https://amzn.to/49A5wjF) book (affiliate link)
--- [Designing Data Intensive Applications] (https://amzn.to/3JgCZFh) book (affiliate link)
The intro and outro music is from [The Hug] (http://freemusicarchive.org/music/The_Freak_Fandango_Orchestra/Love_death_and_a_drunken_monkey/04_-_The_Hug) by [The Freak Fandango Orchestra] (http://freemusicarchive.org/music/The_Freak_Fandango_Orchestra/) / [CC BY-SA] (http://creativecommons.org/licenses/by-sa/3.0/)
Sponsored By:
--- [Starburst] (https://www.dataengineeringpodcast.com/starburst) : [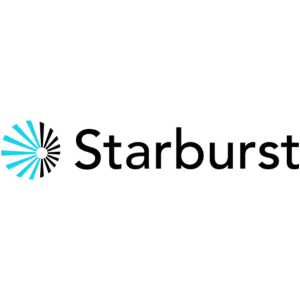 This episode is brought to you by Starburst - a data lake analytics platform for data engineers who are battling to build and scale high quality data pipelines on the data lake. Powered by Trino, Starburst runs petabyte-scale SQL analytics fast at a fraction of the cost of traditional methods, helping you meet all your data needs ranging from AI/ML workloads to data applications to complete analytics. Trusted by the teams at Comcast and Doordash, Starburst delivers the adaptability and flexibility a lakehouse ecosystem promises, while providing a single point of access for your data and all your data governance allowing you to discover, transform, govern, and secure all in one place. Starburst does all of this on an open architecture with first-class support for Apache Iceberg, Delta Lake and Hudi, so you always maintain ownership of your data. Want to see Starburst in action? Try Starburst Galaxy today, the easiest and fastest way to get started using Trino, and get $500 of credits free. [dataengineeringpodcast.com/starburst](https://www.dataengineeringpodcast.com/starburst)] (https://www.dataengineeringpodcast.com/starburst)
--- [Datafold] (https://get.datafold.com/replication-de-podcast) : [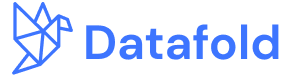 This episode is brought to you by Datafold – a testing automation platform for data engineers that prevents data quality issues from entering every part of your data workflow, from migration to dbt deployment. Datafold has recently launched data replication testing, providing ongoing validation for source-to-target replication. Leverage Datafold's fast cross-database data diffing and Monitoring to test your replication pipelines automatically and continuously. Validate consistency between source and target at any scale, and receive alerts about any discrepancies. Learn more about Datafold by visiting https://get.datafold.com/replication-de-podcast.] (https://get.datafold.com/replication-de-podcast)
--- [Dagster] (https://dagster.io/lp/dagster-cloud-trial?source=data-eng-podcast) : [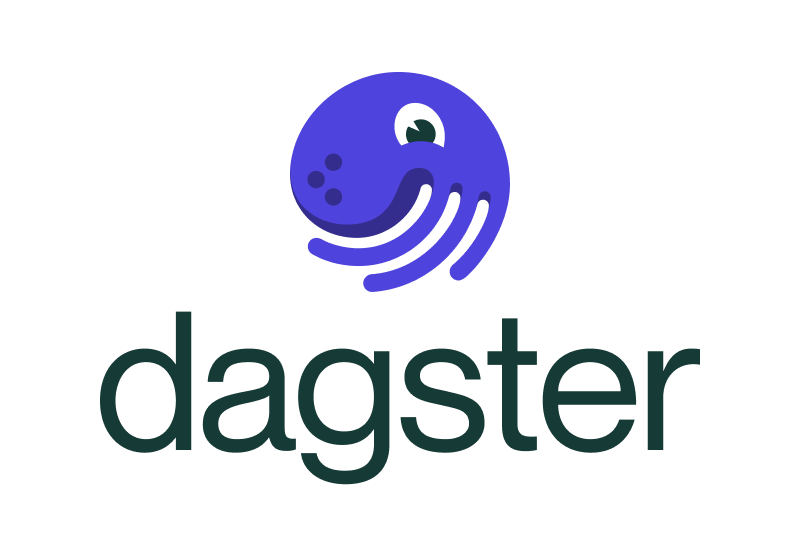 Data teams are tasked with helping organizations deliver on the premise of data, and with ML and AI maturing rapidly, expectations have never been this high. However data engineers are challenged by both technical complexity and organizational complexity, with heterogeneous technologies to adopt, multiple data disciplines converging, legacy systems to support, and costs to manage. Dagster is an open-source orchestration solution that helps data teams reign in this complexity and build data platforms that provide unparalleled observability, and testability, all while fostering collaboration across the enterprise. With enterprise-grade hosting on Dagster Cloud, you gain even more capabilities, adding cost management, security, and CI support to further boost your teams' productivity. Go to [dagster.io](https://dagster.io/lp/dagster-cloud-trial?source=data-eng-podcast) today to get your first 30 days free!] (https://dagster.io/lp/dagster-cloud-trial?source=data-eng-podcast)
[Support Data Engineering Podcast] (https://dataengineering.supercast.com/)
... Read more